Computer Model Reveals How Brain Represents Meaning
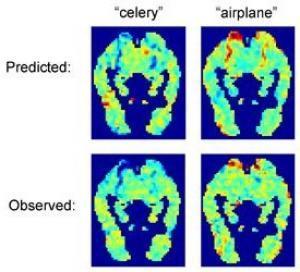
Predicted fMRI images for “celery” and “airplane” show significant similarities with the observed images for each word. Red indicates areas of high activity, blue indicates low activity. (Credit: Illustration courtesy of Science Magazine)
Scientists at Carnegie Mellon University have taken an important step toward understanding how the human brain codes the meanings of words by creating the first computational model that can predict the unique brain activation patterns associated with names for things that you can see, hear, feel, taste or smell.
Researchers previously have shown that they can use functional magnetic resonance imaging (fMRI) to detect which areas of the brain are activated when a person thinks about a specific word. A Carnegie Mellon team has taken the next step by predicting these activation patterns for concrete nouns — things that are experienced through the senses — for which fMRI data does not yet exist.
The work could eventually lead to the use of brain scans to identify thoughts and could have applications in the study of autism, disorders of thought such as paranoid schizophrenia, and semantic dementias such as Pick’s disease.
The team, led by computer scientist Tom M. Mitchell and cognitive neuroscientist Marcel Just, constructed the computational model by using fMRI activation patterns for 60 concrete nouns and by statistically analyzing a set of texts totaling more than a trillion words, called a text corpus. The computer model combines this information about how words are used in text to predict the activation patterns for thousands of concrete nouns contained in the text corpus with accuracies significantly greater than chance.
The findings are being published in the May 30 issue of the journal Science.
“We believe we have identified a number of the basic building blocks that the brain uses to represent meaning,” said Mitchell, who heads the School of Computer Science’s Machine Learning Department. “Coupled with computational methods that capture the meaning of a word by how it is used in text files, these building blocks can be assembled to predict neural activation patterns for any concrete noun. And we have found that these predictions are quite accurate for words where fMRI data is available to test them.”
Just, a professor of psychology who directs the Center for Cognitive Brain Imaging, said the computational model provides insight into the nature of human thought. “We are fundamentally perceivers and actors,” he said. “So the brain represents the meaning of a concrete noun in areas of the brain associated with how people sense it or manipulate it. The meaning of an apple, for instance, is represented in brain areas responsible for tasting, for smelling, for chewing. An apple is what you do with it. Our work is a small but important step in breaking the brain’s code.”
In addition to representations in these sensory-motor areas of the brain, the Carnegie Mellon researchers found significant activation in other areas, including frontal areas associated with planning functions and long-term memory. When someone thinks of an apple, for instance, this might trigger memories of the last time the person ate an apple, or initiate thoughts about how to obtain an apple.
“This suggests a theory of meaning based on brain function,” Just added.
In the study, nine subjects underwent fMRI scans while concentrating on 60 stimulus nouns — five words in each of 12 semantic categories including animals, body parts, buildings, clothing, insects, vehicles and vegetables.
To construct their computational model, the researchers used machine learning techniques to analyze the nouns in a trillion-word text corpus that reflects typical English word usage. For each noun, they calculated how frequently it co-occurs in the text with each of 25 verbs associated with sensory-motor functions, including see, hear, listen, taste, smell, eat, push, drive and lift. Computational linguists routinely do this statistical analysis as a means of characterizing the use of words.
These 25 verbs appear to be basic building blocks the brain uses for representing meaning, Mitchell said.
By using this statistical information to analyze the fMRI activation patterns gathered for each of the 60 stimulus nouns, they were able to determine how each co-occurrence with one of the 25 verbs affected the activation of each voxel, or 3-D volume element, within the fMRI brain scans.
To predict the fMRI activation pattern for any concrete noun within the text corpus, the computational model determines the noun’s co-occurrences within the text with the 25 verbs and builds an activation map based on how those co-occurrences affect each voxel.
In tests, a separate computational model was trained for each of the nine research subjects using 58 of the 60 stimulus nouns and their associated activation patterns. The model was then used to predict the activation patterns for the remaining two nouns. For the nine participants, the model had a mean accuracy of 77 percent in matching the predicted activation patterns to the ones observed in the participants’ brains.
The model proved capable of predicting activation patterns even in semantic areas for which it was untrained. In tests, the model was retrained with words from all but two of the 12 semantic categories from which the 60 words were drawn, and then tested with stimulus nouns from the omitted categories. If the categories of vehicles and vegetables were omitted, for instance, the model would be tested with words such as airplane and celery. In these cases, the mean accuracy of the model’s prediction dropped to 70 percent, but was still well above chance (50 percent).
Plans for future work include studying the activation patterns for adjective-noun combinations, prepositional phrases and simple sentences. The team also plans to study how the brain represents abstract nouns and concepts.
The Carnegie Mellon team included Andrew Carlson, a Ph.D. student in the Machine Learning Department; Kai-Min Chang, a Ph.D. student in the Language Technologies Institute; and Robert A. Mason, a post-doctoral fellow in the Department of Psychology. Others are Svetlana V. Shinkareva, now a faculty member at the University of South Carolina, and Vicente L. Malave, now a graduate student at the University of California, San Diego. The research was funded by grants from the W.M. Keck Foundation and the National Science Foundation.